FIAS scientists employ machine learning to investigate thunderstorms.
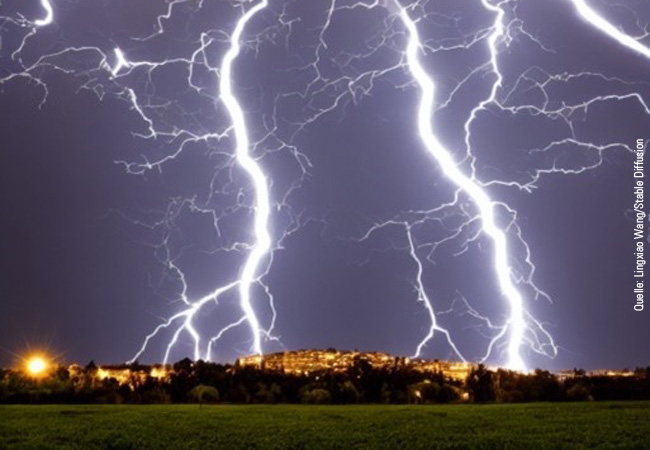
Each year, lightning strikes almost half a million times in Germany alone. Lasting for only fractions of a second, the details of these fascinating lightning phenomena are difficult to see with optical equipment, much less so with the naked eye. Not surprisingly, up to now the investigation of lightning has been not only very time-consuming, it also requires a lot of experience. Using carefully selected algorithms and Artificial Intelligence, researchers at the Frankfurt Institute for Advanced Studies (FIAS) have now succeeded in resolving lightning structures faster and in much more detail.
Aiding the scientists in their work is the Dutch-German radio telescope LOFAR (Low Frequency Array), a European network of many individual antenna stations resembling solar panels. Each of these flat stations measures radio waves with wavelengths ranging from 1 to 10 meters, which we can neither see nor hear – similar to those used to transmit radio programs. The LOFAR network of telescopes was constructed and expanded during the last decade, and is used to research cosmic events and astronomical data. LOFAR registers flashes of lightning as disruptions, which it generally records unintentionally alongside other events.
The very sensitive antennas continuously collect extremely large volumes of data, which cannot be used in their original format and instead have to be pared down to their informative details. This is where scientists at the University of Groningen (The Netherlands) come into play: They process the raw data recorded by LOFAR into a format FIAS can use. At this point, the working group led by FIAS fellow Kai Zhou can put its strengths into play: its specialty lies in Deep Learning, a branch of machine learning that resolves enormous quantities of data with a high degree of abstraction, allowing it to be understood and represented in a useful manner.
Better assessment of dangers
Together with Zhou and co-workers Brian Hare, Horst Stöcker and Olaf Scholten, Lingxiao Wang, a postdoc in the working group, has developed a new method for using algorithms from machine learning and correlation analyses to identify structures in lightning data. “The exploration of the lightning structure is the first step towards understanding the occurrence and evolution of lightning phenomena,” Wang explains. “If we can improve our understanding of these extreme phenomena, we will be better able in future to assess and avoid the dangers caused by lightning strikes.”
In a recent study published in the journal Chaos, Solitons & Fractals, the team sheds light on the understanding of lightning structures and provides insights into the complex correlation functions for different lightning phenomena. Using machine-learning algorithms, they help identify structures with the aid of numerous spatio-temporal points in a high dimensional space – which would be very time-consuming to do with the naked eye. This new method is a powerful tool for combing vast multidimensional data sets for unique structures.
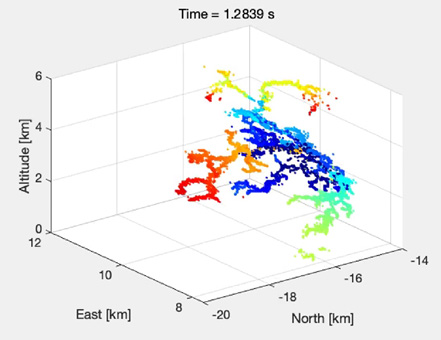
Detail from an animation of a lightning event in a space of 4 x 6 x 6 kilometers over a period of 0.13 seconds. Displayed in blue are early initiation points, which propagate within fractions of a second through the regions shown in green and yellow, followed by red. Images like this can be created thanks to the processing of large volumes of LOFAR data.
Source: Lingxiao Wang/FIAS
Wang’s everyday work takes place at a computer screen. He develops algorithms, or, put in simpler terms, well-defined calculation steps, which he combines to obtain the best reproduction of the existing data while simultaneously reducing it to the most important aspects. This process clarifies the physics behind lightning, for example. “One of our algorithms can simplify high dimensional data down to a two-dimensional representation,” says Wang. This allows him to present a mass of jumbled data on paper, where it can be further interpreted. Within this reduced space, another algorithm then draws together similar data points on the basis of their proximity. These machine-learning algorithms are a type of Artificial Intelligence, i.e. they are capable of learning from the data and thus improving their performance over time.
“A typical lightning phenomenon that we analyze has four dimensions: length, width, height and time,” Wang explains. This information yields a spatial image that spans several kilometers and whose development can be tracked through time – whereby in this case, “time” means only a fraction of a second, which is shown in a decelerated manner and divided into individual steps. By applying their algorithms to lightning data, the researchers are able to recognize several different structures within flashes of lightning. Much like a tree, a bolt of lightning consists of main branches with innumerable smaller branches right down to fine side-branches known as “needles”. In addition, on the basis of the resolved data, the charge properties, fine channel structures and many other physical details can now be determined “more or less at the push of a button,” Wang says. The automatic analysis of lightning data accelerates the evaluation process conducted by extremely experienced scientists – which has until now been both highly complex and time-consuming –, cutting the time needed down from several months to just a few minutes.
Climate change could alter lightning frequency
The analyses performed by Wang and his colleagues are creating the foundation for a closer investigation of the effects lightning has on human beings and the environment. As such, lightning sometimes causes forest fires – and the risk may well increase in future during prolonged periods of drought. At the same time, lightning flashes also reflect changes in the atmosphere – as a result of climate change, their frequency and structures could alter as well. The new analytical method allows researchers to observe precisely this development. A better understanding of lightning structures will also enable us to better gauge their effects – on air travel, for instance.
The next thing Wang wants to examine is how the clusters extracted by the algorithm develop. He suspects that the temporal development of different lightning phenomena follows certain rules. This practical investigation is the next step toward developing an understanding of the occurrence of lightning with the aid of machine learning.
The combination of algorithms used in this study can also be applied in other fields, where the search for unique structures in huge multi-dimensional data sets plays a central role, including the analysis and prediction of earthquakes, traffic flows in urban centers, or gene sequences, for instance.
Anja Störiko
Publications
Lingxiao Wang, Brian M. Hare, Kai Zhou, Horst Stöcker und Olaf Scholten
Identifying Lightning Structures via Machine Learning
Chaos, Solitons & Fractals 170, 113346 (2023)
Brian M. Hare, Olaf Scholten, Joseph Dwyer et al.
Needle-like structures discovered on positively charged lightning branches
Nature, 2019, 568 (7752): 360-363